Youtube recommendations are already online RL, is intensive learning far from a large-scale application in the recommended advertising industry?
The following articles are derived from the Deep Transfer Gate , the author depth transmitter door
Focus on depth recommendation systems and CTR estimates
Introduction: This article is the sixth article in the "Deep Recommendation Systems" column, and this series will introduce the most cutting-edge changes to the recommendation system industry, driven by the power of deep learning. Based on the latest papers of several summits in 2019, this paper summarizes the latest developments that deep intensive learning has brought to the recommendation system and CTR's estimated industry.
Welcome to reprint, reprint please indicate the source and link, more about the depth of the recommendation system quality content please pay attention to the following channels.
Know the column: deep recommendation system
Weibo: Deep Transfer Gate
Public Number: Deep Transfer Gate
Any Google product, must be a boutique. Think of that year (in fact, near 2016), YoutubeDNN and WDL's out-of-the-sky lead the industry trend of recommendation systems and CTR estimation so far, setting off a wave of large-scale elegant and efficient upgrading of deep learning models by recall and sort layer algorithms. Development has in fact formed a large family group of industry recommendation systems and advertising CTR estimates, as detailed in the previous article of the family map.Latest! Top 5 2019 must-read in-depth recommendation system-related papers
Of course, the focus of this article is not to look back. The good man does not mention the brave, but based on the current look at the next recommendation system and CTR estimates where the industry's road is. The reason for this is that Google has published industry-style application-enhanced learning papers in WSDM 2019 and IJCAI 2019, and claims to have made significant gains from the deep learning models already available online in the online experiments at the Youtube recommended sorting layer. Therefore, this paper summarizes the latest developments in the industry in the recommendation system and CTR estimation of intensive learning applications at several summits 2019, and welcomes your experienced peers to exchange more and make common progress.
As we all know, although intensive learning in The Go, games and other fields, but in the recommendation system and CTR estimation application has been a lot of difficulties have not been resolved. On the one hand, because the exploration of the combination of intensive learning and recommendation system has just begun, the current program has not been as effective as the traditional machine learning upgrade deep learning, upgrading intensive learning in the effect of the relatively existing deep learning model is not yet able to make a qualitative leap; This results in the current application of enhanced learning models in industry is not cost-effective. Embarrassingly, many papers use Baseline when upgrading RL comparisons to traditional machine learning algorithms rather than the latest deep learning models, which are hard to believe to some extent.
So the emergence of Google's two enhanced learning applications for YouTube recommendations has given us some exciting hope. First, Baseline, which claims to compare effects, is the latest deep learning model on YouTube's recommended line; And one of the paper's authors, Minmin Chen, said on Industry Day that online experiments showed the biggest increase in YouTube's single project in nearly two years. This does not mean that the combination of intensive learning and recommendation systems has matured, at least to give everyone some motivation to actively try in the industry.
Top-K Off-Policy Correction for a REINFORCE Recommender System,WSDM 2019
The main highlight of this paper is to propose an Off-Policy fix for Top-K to apply the Polity-Gradient algorithm in RL to millions of Youtube online recommendation systems in the motion space.
As we all know, Youtube's recommended system architecture is divided into two main layers: recall and sorting. The algorithms in this article are applied on the recall side. Modeling ideas are similar to RNN recalls, given a user's behavior history, predicting the user's next click. Due to the complexity of the On-Policy approach to the system training architecture, this article turns to Off-Policy's training strategy. This means that instead of real-time policy updates based on user interactions, model training is based on user feedback collected into the logs.
This Off-Policy approach can cause problems for model training in the Polity-Gradient class, with policy gradients calculated by different policys on the one hand, and data on other recall strategies collected by the same user's behavior history on the other. Therefore, an Off-Policy correction scheme based on value weighting is proposed, and a first-order approximate induction is made for the calculation of strategy gradient.
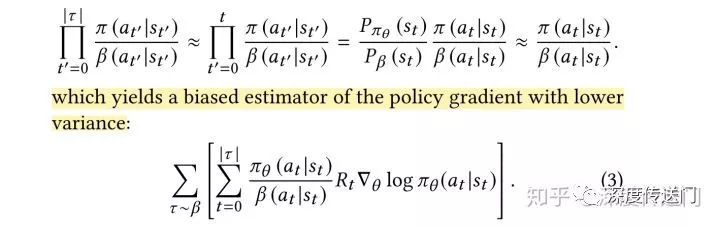
Because the next user click is predicted based on the user's interaction history, RNN is also used to model the user's State transformation. The RNN units, including LSTM and GRU, were mentioned in the paper, and it was found that The RNN units of Chaos Free worked best because they were stable and efficient.
In the above-mentioned strategy correction formula (3) the most difficult to obtain is the user's behavior strategy, ideally, when collecting logs at the same time the user's corresponding user policy is the click probability to collect down, but due to objective reasons such as different policies in the text for the user's behavior strategy to use another set of parameters to estimate, and prevent its gradient back to affect the training of the main RNN network.
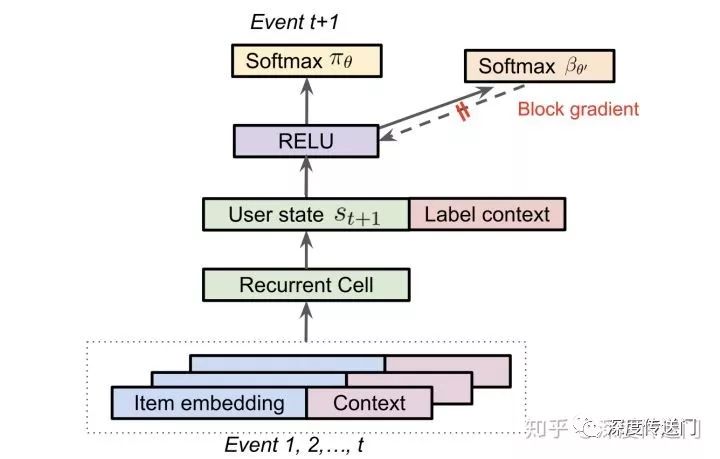
In addition, because in the recommendation system, the user can see k candidates presented to the user at the same time, the user may interact with multiple items presented at the same time. Therefore, you need to extend the policy to predict the top-Kitem that the user might click next time based on the user's behavior history.
Assuming that the show K non-repeating item's reward is equal to the total of each item's reward, according to the formula we can get Top-K's Off-Policy correction strategy gradient as follows, compared with the above Top 1 correction formula is mainly an increase of one factor containing K. That is, as K grows, the strategy gradient drops to 0 faster than the original formula.
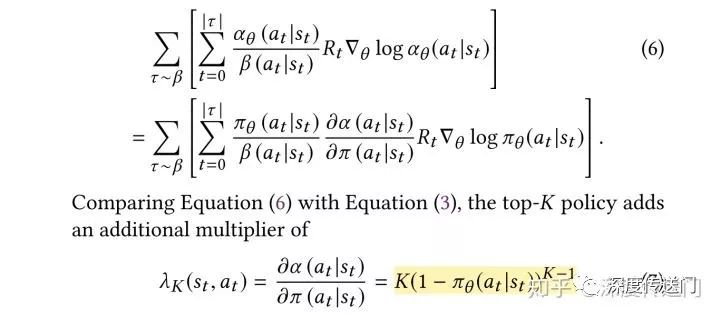
From the point of view of experimental results, a series of experiments were carried out to compare and verify the effect, in which Top-K's Off-Policy correction scheme brought about an increase of 0.85% of the playback time on the line. And as mentioned earlier, Minmin Chen also mentioned on Industry Day that online experiments showed the biggest growth in YouTube's single project in nearly two years.
In addition, in the latest issue of Google AI Blog, a classification based on enhanced learning Of-Policy was announced to predict which machine learning model would produce the best results. Interested can continue to extend the reading.
Reinforcement Learning for Slate-based Recommender Systems: A Tractable Decomposition and Practical Methodology,IJCAI 2019
This article is a little later than the first article, and the proposed methods are not the same, but similar claims have been made on the Youtube online recommendation system has achieved good results. The main contribution is to propose a Q-Learning algorithm called SLATEQ to optimize the long-term benefits of LTV (Long-term Value) in the recommendation system that simultaneously presents multiple item situations to the user.
First of all, this article is different from the first article, first of all, the first article assumes that in the recommendation system at the same time show K non-repeatitem (this article is called Slate) reward reward equal to the total of each item's reward, which is considered unreasonable in this article, so modeling slate's LTV and individual item's LTV relationship;
From a system architecture perspective, this article expands Youtube's existing real-time-only rangeker, which is multi-target forward-depth e-learning for indicators such as CTR and long-term earnings LTV. It's worth noting that in order to ensure the fairness of the online experiment, there are all the same features and network parameters on Youtube, except for the multi-target.
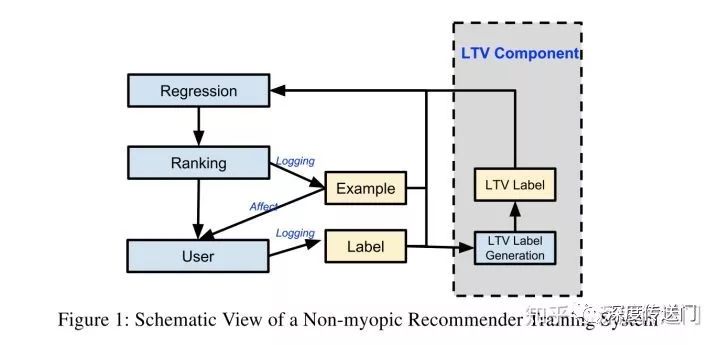
In the final experimental section, the evaluation in this article is User engagement, and you can see from the following image that the effect improvement is obvious and stable.
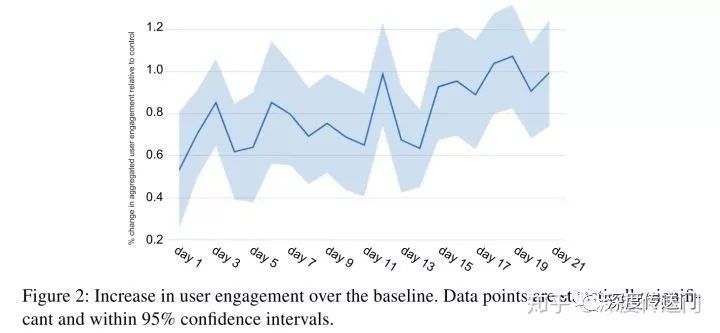
Other industry developments
In addition to Google's two papers above, other companies in the industry are actively trying to strengthen learning in the recommendation system, and here's a brief list of some of the progress so far:
Generative Adversarial User Model for Reinforcement Learning Based Recommendation System,ICML 2019
In this paper, which was received by ICML 2019, the authors propose to use the generation of anti-user models as a simulation environment for intensive learning, first in this simulation environment for offline training, and then based on online user feedback for immediate policy updates, thereby greatly reducing the demand for online training samples. In addition, the authors suggest recommendations in sets rather than individual items, and uses Cascading-DQN's neural network structure to solve the problem that the combined recommendation strategy search space is too large.
Virtual-Taobao: Virtualizing real-world online retail environment for reinforcement learning,AAAI 2019
Ali at AAAI 2019, a "virtual Taobao" simulator that uses RL and GAN to plan the best product search display strategy, increases Taobao's revenue by 2% in the real world. What is lacking in the U.S. and China is that baseline is still a traditional supervised learning rather than a deep learning program.
Large-scale Interactive Recommendation with Tree-structured Policy Gradient,AAAI 2019
Bibliography
[1] Deep Neural Networks for YouTube Recommendations, RecSys 2016
[2] Wide & Deep Learning for Recommender Systems,
[3] ai.googleblog.com/2019/
[4] Reinforcement Learning to Optimize Long-term User Engagement in Recommender Systems
Ali's "Enhanced Learning in Ali's Technological Evolution and Business Innovation"
[6] 京东的 Deep Reinforcement Learning for Page-wise Recommendations
Related articles:
The recommended recall algorithm is a deep recall model
Bird gun for gun, how to use ai Lab open source Chinese the power of word vectors?
Read the AutoEncoder model evolution map in a text
Latest! The five top 2019 must-read in-depth recommendation system and CTR estimates related papers
Read the GAN evolution map in a text
Go to "Discovery" - "Take a look" browse "Friends are watching"