Why did Google create ghost ads?
Everyone must know about advertising, but what is a ghost ad? In fact, ghost ads are in English, derived from a Google paper by Johnson, Garrett A., Randall A. Lewis, and Elmar I. Nubbemeyer. "Ghost Ads: Improving the Economics of Measuring Online Ad Effectiveness." (2016)。 Ghost ads are a system they created to improve the effectiveness of online advertising. Because some ads are implemented as ghosts that users can't see, it's called a "ghost ad" system, dedicated to randomized controlled experiments for online advertising.
Gardeners have written a lot of introductions to online experiments on and off, but most of them don't involve online advertising. Perhaps you will think, online advertising and other online experiments are not the same, what need special attention? The gardener read the paper in a fog before listening to Randall, and he didn't know what to do. After listening to Randall, I probably understood the subtleties. Until recently I began to do advertising-related things, seriously really turned out this paper, read through, by the way to share with you from the beginning to the present some insights.
The big background of online advertising
Before detailing ghost advertising, the gardener gives a rough look at online advertising. The big profits for user-facing Internet companies are three: advertising, games, selling things (physical products and services). Most websites are free for users, so the way to make money comes from advertising, such as Google and Baidu. Simple as a paid search, that is, the relevant website pays Google, so that users can search for specific keywords, you can see their own website first. Of course, it's more common to have a variety of pictures and videos, such as forcing a short ad to be inserted during Youku's chase, or brushing Weibo to find that the time stream is filled with less familiar bloggers. For the average user, you may not think about the complex ecosystem behind the ads you see. The gardener didn't know until he saw the picture. Well, it turns out that the ecological chain can be so long.
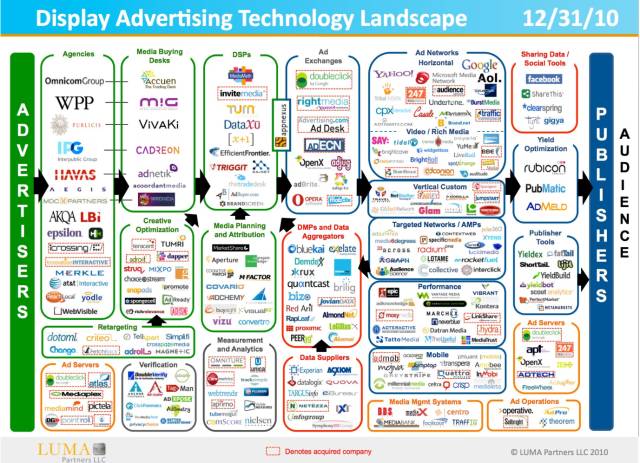
Source: http://earlh.com/blog/2011/07/19/online-display-advertising-ecosystem/
Apparently paying advertisers in this ecosystem want to know where they spend every penny, and it's not worth the bottom. After all, there has always been a famous saying in advertising:
I know that half of my advertising expenses are wasted, but unfortunately, I don't know which half is wasted. (The famous advertising guru John Wernermek, in fact, the gardener did not know how famous he was... )
Therefore, in the advertising ecosystem, measuring the effectiveness of advertising is naturally also an important link. The advantage of online advertising is that you can collect a lot of user feedback data, so that you can better understand where advertisers spend every penny. So it's important for online advertising that we measure the input-output ratio.
The history of the online ad delivery system
Online advertising benefits from the convenience of data tracking, iterating through the first three generations of ad delivery system.
The first generation of ad delivery system
Delivery-based systems (CPM, Cost Per Mille, pay-per-view). Such systems are similar to buying out newspaper pages or television slots, i.e., delivering ads to a pre-agreed quantity, given the advertiser's requirements, regardless of user feedback on the ad. There is no delivery optimization in this situation, and it is completely random based on traffic.
Second-generation ad delivery system
Optimized delivery based on group behavior (CPC, Cost Per Click, pay-per-click, or CPA, Cost Per action, pay-per-view for a given user behavior). Such systems track user feedback data (such as whether to click on an ad, whether to buy an item in an ad), and then return the behavioral data back to the delivery algorithm, specifically for people with good feedback. For example, if I'm going to run a skincare ad that's suitable for the post-80s, the algorithm will be randomly delivered to everyone first, and then when he realizes that feedback after 80 is better, it'll focus on the post-80s user, reducing the ineffective delivery to other users.
Third-generation ad delivery system
Optimized delivery based on individual users. Such systems, based on second-generation systems, add to the consideration of each user's click and behavior history, resulting in more personalized delivery optimization.
The challenge of online ad random control experiments
Compared with ordinary online product experiments, such as testing how layout is better, online advertising experiments will be more complex. In fact, the core question is, if users can not see my ads, then they will buy my products? Some people will, but some may not.
So here's the question, what's the control group for online advertising? If we think about the first generation of advertising systems, then he has a monopoly on delivery. For example, if I buy a banner ad on Sina's home page, everyone who visits Sina's home page sees the same ad. At this point, PSA (public service advertising) is commonly used in advertising as a control group, and then directly compare the difference between the effectiveness of the commercial and public service advertising. However, starting from the second generation system, online advertising began to run based on a variety of machine learning optimization algorithms, so not everyone sees the same ads, naturally actually see the experimental ads of the user and see the public service ads of the user is different. From a causal inference point of view, such a non-random ad delivery can result in a bias in our estimate of the average processing effect of the person being processed (i.e., the person who actually saw the ad) (average treatmenet effect treat oned, some literature is short for ATT). Readers interested in detail can read Imbens, Guido W., and Donald B. Rubin. 23 chapters of causal inference in statistics, social, and biomedical sciences.
Here's a look at the second-generation system optimized with behavioral feedback data to understand why Google's paper started by saying that public service advertising isn't a good control group for second-generation ad delivery.
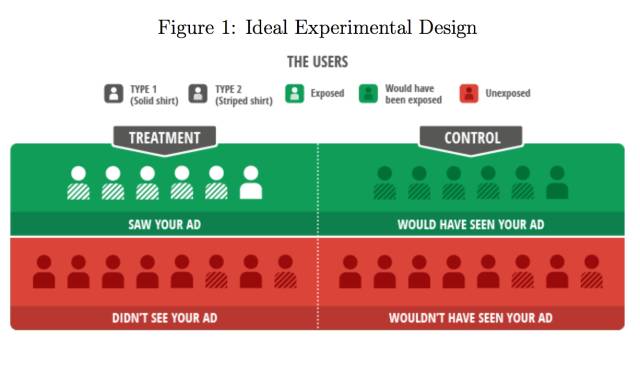
Figure 1, the ideal experimental design. All were randomly assigned to the experimental and control groups, and then we could observe which people in the experimental group actually saw the ads and which of the control groups should have seen the ads if they had been placed in the experimental group. Ideally, the people who saw the ads in the experimental and control groups shown in the green section were the same as the people who should have seen the ads.
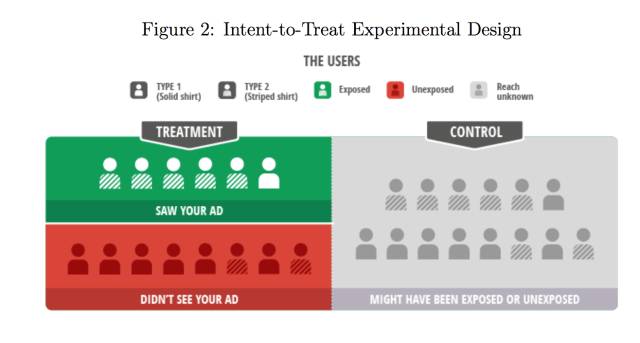
Figure 2 is about intent-to-treat analysis( some of the literature is short for ITT). At this point, we know who in the experimental group saw the ad, but we don't know who in the control group should have seen the ad. This is something we can only analyze according to the initial intent of the experimental design, i.e. go back to the initial phase of randomization, include all the participants in the experiment, and then compare the people in all the experimental and control groups (whether they actually saw the ad or not). Obviously, compared to the ideal scenario in Figure 1, intent processing analysis dilutes the processing effect because it includes people who don't actually see the ads. The advantage of intent processing analysis is that it gives an unethical estimate.
In addition, in real ad delivery practices, estimates of the average effect (i.e. ATT) of the people who see the ads become extra meaningful in the early stages, as it is often the advertising budget that constrains the effectiveness of the ads. Such results can be used to infer what the desired effect can be achieved after the budget increase. And the estimate based on intent processing analysis (ITT) because it does not take into account the increase in the proportion of people who actually see ads as the advertising budget increases, so there is no way to give a better forecast.
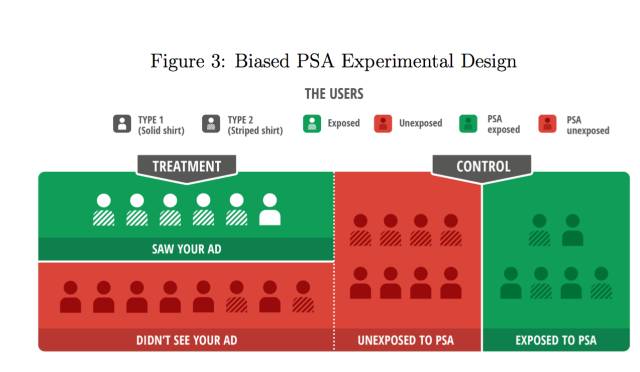
Figure 3 is about the use of public service advertising as a control group. Obviously, because of the existence of the ad delivery optimization system, the people who see ads in the experimental group will see ads in the public service advertising group will not be the same distribution, so if we directly compare the two groups, we will get a biased estimate.
Thus, the goal of ghost advertising is to get something similar to Figure 1 and find a way to observe the average processing effect (ATT) of the person who saw the ad directly from the control group.
The design principle of the ghost advertising system
If we want to get observations consistent with Figure 1, what should the ads that users actually see look like?
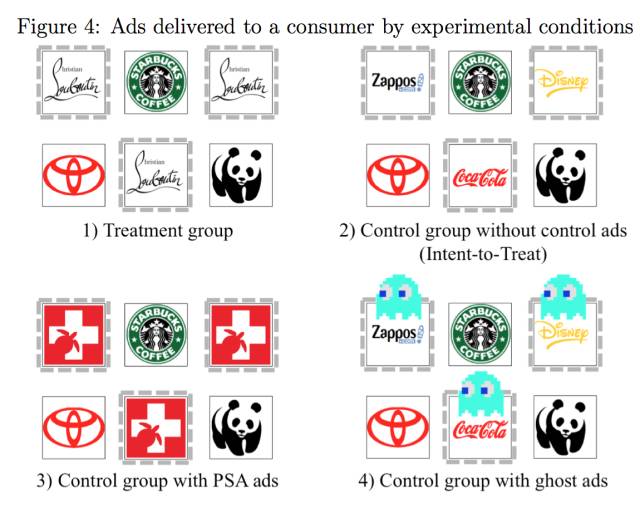
Figure 4 shows the ads that people end up seeing in different situations.
1 in the upper left corner is the experimental group, where three people see the Louboutin shoe ad (grey dotted box), while the remaining three see Toyota, Starbucks and Panda.
The 2 in the upper right corner is the control group, and there are no ads for shoes, so the three people who should have seen the Louboutin shoe ads saw three other ads (another shoe site, Disney and Coca-Cola), but we couldn't tell which three were there. 3 in the lower left corner) is the situation of public service advertising, those three people see is public service advertising. Figure 4 in the lower right corner is the case for ghost ads, and 2) the results are the same, but we know exactly which three people who should have seen the Louboutin ad saw the other ads.
To achieve the results, the Ghost Advertising System needs to add an extra layer of "simulated auctions" to the real ad auction and a step-by-step extra record of the users and behavior of the three people who should have seen the Louboutin ad.
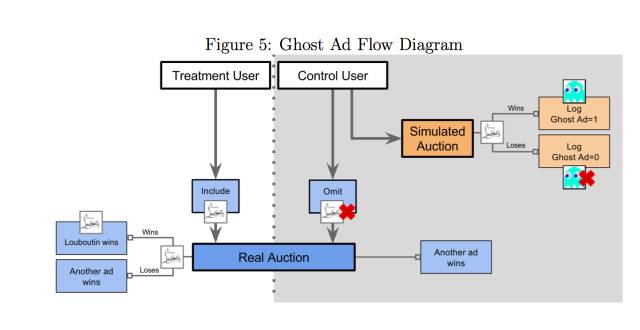
Figure 5 describes how ghost advertising works. On the left are the users of the lab group, and the Louboutin ad is placed in a set of ads that can be displayed, and then goes into the actual ad auction process, where the winning user will see the Louboutin ad. Shown on the right is the situation of the control group. The blue box says that if we take the Louboutin ad directly out of the alternative set, then all users see other ads through the auction system, and we don't know who should have seen the Louboutin ad. So here's an additional yellow box indicating the steps, control group users first go into a "simulated auction" process to decide who should see the Louboutin ads, who should see other ads, and record the results. Then we take out the Louboutin ads, let the other ads go to the actual auction, and record the final results.
So here, the principle of ghost advertising is to use a one-step-in-advance mock auction to have the opportunity to record who should have seen the Louboutin ad, the person in the control group described earlier who should have seen the ad. So even if the second-generation system is optimized for delivery, we still have a plan to record the people who should have seen the ads.
Ghost ads are built on three generations of ad delivery systems
Figure 8 summarizes the effect of ghost ads on three generations of ad delivery systems.
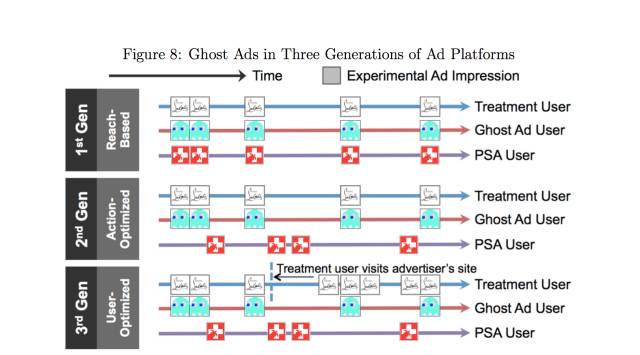
First generation advertising system: The volume-based system does not consider various optimizations based on user feedback, so ghost ads and public service ads will produce the same effect.
Second generation advertising system: behavior-based optimization delivery system will track the user's feedback data, so because of optimization, public service advertising control group will become an invalid control group, and ghost ads can accurately identify the control group of complier.
Third generation advertising system: based on the optimization of individual users. Such systems, based on second-generation systems, add to the consideration of each user's click and behavior history, resulting in a more personalized optimization of delivery. An upgraded version of the ghost ad, called Predicted Ghost Ad Methodology, is needed, and interested readers can read Chapter 4 of the original.
Summarize.
In summary, ghost ads have four advantages over public service ads as a control group:
What is obtained is an unethical estimate
There are no additional execution costs for public service advertising
The correct benchmark success rate
Improved estimation efficiency
But is ghost advertising perfect? In this age of changing online ad optimization technology, ghost advertising has its own limitations. For example, in order to ensure that the effect of the simulated auction and the actual effect of the auction is not very different, ghost advertising needs to be in the stage of the simulated auction to get all the information and data variables that determine whether the actual advertisement is running or not. Once there are other changes in the actual auction process (most commonly budget changes), the simulated auction may be far from the actual effect.
In addition, ghost advertising systems solve the problem of comparing only a single experimental group with a control group, and in practice we often need to compare multiple experimental groups, such as a simple full-factor experiment. Ghost ads don't have a significant advantage over Intent Processing Analysis (ITT) at this point.
Finally, the online advertising experiment is also difficult to escape the common challenge of the general online experiment. For example, most online experiments are still cookie-based, and in the mobile Internet age, it is inevitable that the same user owns multiple devices, and many behaviors, especially multi-step buying behavior, are often not done on one device. Click-based tracking also has its own problems, such as users may see the same ads in multiple channels, such as users buy tickets to book hotels may be in Baidu, Sina, Taobao, Ctrip search, and then think that the final deal by the last click brought about is not fair. These challenges are real, and practitioners have been working to find better measures to achieve more accurate estimates of experimental results.
Review: Xiong Wei, Lang Dawei
Editing by Ray Bowen
Original article, copyrighted.
To inform you of the friendly media, if you need to reproduce, please contact the statistical capital small editor (direct message or send to the mailbox: editor@cos.name), allowed to reproduce in a prominent location please indicate the author and source (reprinted from: statistical capital), andAttach a statistical capital QR code at the end of the article.
Statistics Capital: Professional, human-based, integrity of china's statistical portal.
How to focus: Scan the QR code below. Or find a public account and search for The Capital of Statistics or CapStat.
Past push: Enter the statistical capital session window, click on the upper right corner of the small person icon, view the history message.
The statistical capital welcomes all officials to actively contribute, and the letterbox contact@cos.name
Go to "Discovery" - "Take a look" browse "Friends are watching"